MT that Deceives: Difference between revisions
No edit summary |
No edit summary |
||
Line 1: | Line 1: | ||
Many popular MT systems, such as [http://translate.google.com Google Translate] or [http://www.bing.com/translator/ Bing Translator] (for certain languages), are based purely on statistical models. Such models observe word and phrase co-occurrences in parallel texts and try to learn translation equivalents. | Many popular MT systems, such as [http://translate.google.com Google Translate] or [http://www.bing.com/translator/ Bing Translator] (for certain languages), are based purely on statistical models. Such models observe word and phrase co-occurrences in parallel texts and try to learn translation equivalents. | ||
In some cases, this approach leads to '''systematic errors'''. | [[File:nemam_kocku.png|thumb|300px|'''Example of an error during phrase extraction.''' The system learns a translation pair ''"nemám" = "I have"'' which has the opposite meaning.]] | ||
In some cases, this approach leads to '''systematic errors'''. The picture illustrates a common issue with negation -- in many languages (such as Czech), negation is expressed by a prefix ("''ne''" in this case). Moreover, Czech uses double negatives: the sentence ''Nemám žádnou kočku.'' corresponds to English ''I_do_not_have no cat.'' word by word. Therefore the automatic procedure learns a wrong translation rule ''I have''=''nemám''. Whenever this rule is applied, the meaning of the translation is completely reversed. | |||
Other examples of notorious errors include named entities, such as: | |||
''Jan Novák potkal Karla Poláka. -> John Smith met Charles Pole.'' |
Revision as of 15:14, 5 November 2014
Many popular MT systems, such as Google Translate or Bing Translator (for certain languages), are based purely on statistical models. Such models observe word and phrase co-occurrences in parallel texts and try to learn translation equivalents.
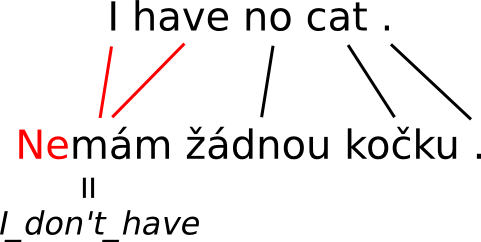
In some cases, this approach leads to systematic errors. The picture illustrates a common issue with negation -- in many languages (such as Czech), negation is expressed by a prefix ("ne" in this case). Moreover, Czech uses double negatives: the sentence Nemám žádnou kočku. corresponds to English I_do_not_have no cat. word by word. Therefore the automatic procedure learns a wrong translation rule I have=nemám. Whenever this rule is applied, the meaning of the translation is completely reversed.
Other examples of notorious errors include named entities, such as:
Jan Novák potkal Karla Poláka. -> John Smith met Charles Pole.